import torch
import torch.nn as nn
import torch.nn.functional as F
import torch.optim as optim
from torch.autograd import Variable
import matplotlib.pyplot as plt
from torchvision import datasets, transforms # datasets包含常用的数据集,transform 对图像进行预处理
import cv2
# training settings
batch_size = 64
train_dataset = datasets.MNIST(root='./dataset/mnist',train=True,transform=transforms.ToTensor(),download=True)
test_dataset = datasets.MNIST(root='./dataset/mnist',train=False,transform=transforms.ToTensor())
# Data Loader(Input Pipeline)是一个迭代器,torch.utils.data.DataLoader作用就是随机的在样本中选取数据组成一个小的batch。shuffle决定数据是否打乱
train_loader = torch.utils.data.DataLoader(dataset=train_dataset,batch_size=batch_size,shuffle=True)
test_loader = torch.utils.data.DataLoader(dataset=test_dataset,batch_size=batch_size,shuffle=False)
# 可视化数据图像
for i in range(1):
plt.figure()
print(train_loader.dataset.train_data[i].shape)
plt.imshow(train_loader.dataset.train_data[i].numpy())
torch.Size([28, 28])

x = torch.randn(2, 2, 2)
# firstly change the data into diresed dimension, then reshape the tensor according to what I want
x.view(-1, 1, 4)
# 理解迭代器的深层含义,torch.utils.data.DataLoader的作用理解
for (data, target) in train_loader:
for i in range(1):
plt.figure()
print("target:",target[i])
print("data:",data.shape)
plt.imshow(data[i].numpy()[0])
break
target: tensor(3)
data: torch.Size([64, 1, 28, 28])
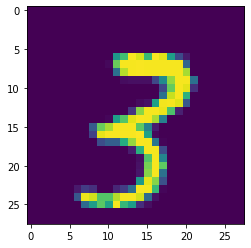
class LeNet5(nn.Module):
def __init__(self):
super(LeNet5, self).__init__()
self.conv1 = nn.Conv2d(1, 6, 5, 1, 2)
self.pool = nn.MaxPool2d(2, 2)
self.conv2 = nn.Conv2d(6, 16, 5)
self.fc1 = nn.Linear(16 * 5 * 5, 120)
self.fc2 = nn.Linear(120, 84)
self.fc3 = nn.Linear(84, 10)
def forward(self, x):
x = self.pool(F.relu(self.conv1(x)))
x = self.pool(F.relu(self.conv2(x)))
x = x.view(x.size()[0], -1)
x = F.relu(self.fc1(x))
x = F.relu(self.fc2(x))
x = self.fc3(x)
return x
input1 = torch.rand([64,1,28,28])
model = LeNet5()#实例化
print(model)
output = model(input1)
print(output.shape)
LeNet5(
(conv1): Conv2d(1, 6, kernel_size=(5, 5), stride=(1, 1), padding=(2, 2))
(pool): MaxPool2d(kernel_size=2, stride=2, padding=0, dilation=1, ceil_mode=False)
(conv2): Conv2d(6, 16, kernel_size=(5, 5), stride=(1, 1))
(fc1): Linear(in_features=400, out_features=120, bias=True)
(fc2): Linear(in_features=120, out_features=84, bias=True)
(fc3): Linear(in_features=84, out_features=10, bias=True)
)
torch.Size([64, 10])
model = LeNet5()
optimizer = torch.optim.SGD(model.parameters(), lr = 0.1, momentum=0.9)
loss_func = torch.nn.CrossEntropyLoss()
DEVICE = torch.device("cuda" if torch.cuda.is_available() else "cpu")
def train(model, device, train_loader, optimizer, epoch):
model.train()
for batch_idx, (data, target) in enumerate(train_loader):
data, target = data.to(device), target.to(device)
optimizer.zero_grad()
output = model(data)
loss = loss_func(output, target)
loss.backward()
optimizer.step()
if (batch_idx + 1) % 30 == 0:
print('Train Epoch: {} [{}/{} ({:.0f}%)]\tLoss: {:.6f}'.format(
epoch, batch_idx * len(data), len(train_loader.dataset),
100. * batch_idx / len(train_loader), loss.item()))
def test(model, device, test_loader):
model.eval()
test_loss = 0
correct = 0
with torch.no_grad():
for data, target in test_loader:
data, target = data.to(device), target.to(device)
output = model(data)
test_loss += loss_func(output, target) # 将一批的损失相加
pred = output.max(1, keepdim=True)[1] # 找到概率最大的下标
correct += pred.eq(target.view_as(pred)).sum().item()
test_loss /= len(test_loader.dataset)
print("\nTest set: Average loss: {:.4f}, Accuracy: {}/{} ({:.0f}%) \n".format(
test_loss, correct, len(test_loader.dataset),
100. * correct / len(test_loader.dataset)
))
for epoch in range(1, 2):
train(model, DEVICE, train_loader, optimizer, epoch)
test(model, DEVICE, test_loader)
torch.save(model, 'LeNet5model.pth')
Train Epoch: 1 [1856/60000 (3%)] Loss: 2.201183
Train Epoch: 1 [3776/60000 (6%)] Loss: 1.017704
Train Epoch: 1 [5696/60000 (9%)] Loss: 0.476295
Train Epoch: 1 [7616/60000 (13%)] Loss: 0.209916
Train Epoch: 1 [9536/60000 (16%)] Loss: 0.305845
Train Epoch: 1 [11456/60000 (19%)] Loss: 0.212837
Train Epoch: 1 [13376/60000 (22%)] Loss: 0.563381
Train Epoch: 1 [15296/60000 (25%)] Loss: 0.197010
Train Epoch: 1 [17216/60000 (29%)] Loss: 0.401028
Train Epoch: 1 [19136/60000 (32%)] Loss: 0.279738
Train Epoch: 1 [21056/60000 (35%)] Loss: 0.105045
Train Epoch: 1 [22976/60000 (38%)] Loss: 0.032418
Train Epoch: 1 [24896/60000 (41%)] Loss: 0.401644
Train Epoch: 1 [26816/60000 (45%)] Loss: 0.267381
Train Epoch: 1 [28736/60000 (48%)] Loss: 0.237284
Train Epoch: 1 [30656/60000 (51%)] Loss: 0.114824
Train Epoch: 1 [32576/60000 (54%)] Loss: 0.027149
Train Epoch: 1 [34496/60000 (57%)] Loss: 0.183956
Train Epoch: 1 [36416/60000 (61%)] Loss: 0.229391
Train Epoch: 1 [38336/60000 (64%)] Loss: 0.227810
Train Epoch: 1 [40256/60000 (67%)] Loss: 0.028918
Train Epoch: 1 [42176/60000 (70%)] Loss: 0.078373
Train Epoch: 1 [44096/60000 (73%)] Loss: 0.182829
Train Epoch: 1 [46016/60000 (77%)] Loss: 0.105499
Train Epoch: 1 [47936/60000 (80%)] Loss: 0.065697
Train Epoch: 1 [49856/60000 (83%)] Loss: 0.035855
Train Epoch: 1 [51776/60000 (86%)] Loss: 0.144723
Train Epoch: 1 [53696/60000 (89%)] Loss: 0.184232
Train Epoch: 1 [55616/60000 (93%)] Loss: 0.157933
Train Epoch: 1 [57536/60000 (96%)] Loss: 0.183763
Train Epoch: 1 [59456/60000 (99%)] Loss: 0.097586
Test set: Average loss: 0.0018, Accuracy: 9706/10000 (97%)
使用自己的图像进行测试:
three=cv2.imread("dataset/3.png",cv2.IMREAD_GRAYSCALE)
three=cv2.resize(three,(28,28))
print(three.shape)
plt.imshow(three,cmap='gray')
plt.show()
#three=three.flatten()
print(three.shape)
(28, 28)
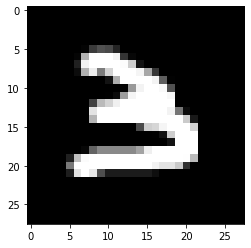
three=three.reshape((1,1,28,28))
three=torch.from_numpy(three)
three=three.float()
print(three.shape)
out1 = model(three) # outputs,out1修改为你的网络的输出
print(out1)
pred = out1.max(1, keepdim=True)[1]
print("该图片中的数字为:",pred.squeeze().item())#squeeze()给tensor降维
torch.Size([1, 1, 28, 28])
tensor([[-246.1170, -636.9862, 64.5719, 1379.5076, -142.7319, 364.0976,
-389.4799, -231.8329, -188.2358, 24.6477]], grad_fn=<AddmmBackward>)
该图片中的数字为: 3
打开App,阅读手记